Protecting Data in Clinical Trial Disclosure: Best Practices in Personal Data Anonymization
Article
Regulatory & Compliance
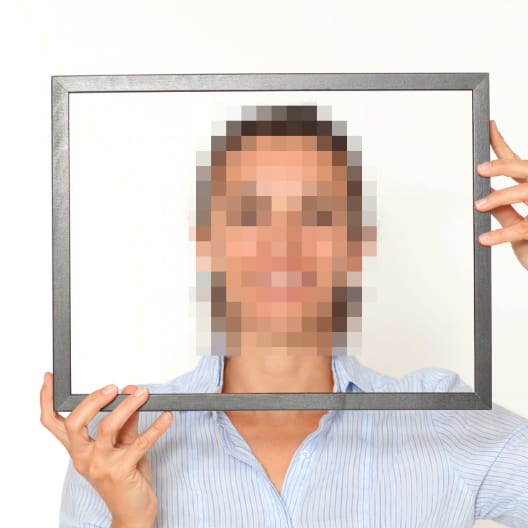
As of January 2023, the European Medicines Agency (EMA) is requiring all newly initiated clinical trials to be registered through its Clinical Trials Information System (CTIS), replacing the EU Clinical Trials Registry.1 This means regulatory submission, authorization, and supervision of clinical trials in the European Union and the European Economic Area must be done through CTIS.2
In addition, the EMA relaunched the Policy on the Publication of Clinical Data for Medicinal Products for Human Use (Policy 0070) in September 2023 with the goal of increasing transparency and promoting the sharing of clinical trial data to benefit health for all.
As part of CTIS and Policy 0070, marketing authorization holders (MAHs) are required to anonymize all personal data that could identify patients participating in the trial. In the recent Citeline webinar, “How to Protect Sensitive Data in Public Clinical Trial Disclosures: Industry Insights and Best Practices for Data Anonymization and CCI Redaction,” panelists discussed best practices.
Elliot Zimmerman, CEO, Real Life Sciences, says anonymization techniques can differ depending on the personal data being anonymized. Such attributes include age, gender, body mass index, height, and race, and direct identifiers like subject IDs and phone numbers.
“Anonymization, when done in a quantitative manner, will evaluate all of those attributes and make decisions for each one respectively based on the entire dataset and based on the entire subject population for the trial,” Zimmerman says. “And that’s really what we’re talking about here in terms of determining what is the best way to anonymize with considering all the quasi-identifiers and direct identifiers and managing to a specific risk threshold, and at the same time trying to maximize the ability of the data for the person the other end that’s actually going to be reviewing this and making use of it.”
“If you look at the de-identification methods under HIPAA, for example, the expert determination method is about engaging a statistician to consider whether certain information, whether alone or in combination with other available information, would potentially identify an individual and the level of risk presented by this” says Peter Vine, Head of Privacy at Norstella. “It’s really worth thinking about direct versus indirect identifiers, the type of data in scope of anonymization and what methodology and anonymization standard pursuant to the relevant law or regulation in the specific region you are looking to report on.”
Zimmerman says he believes regulators prefer retention of certain identifiers and suppressing or increasing generalization of other identifiers. “The term suppression often is synonymous with redaction,” he says. “So, for these quasi-identifiers that are really important, like age, body mass index, gender, race, et cetera, they will want as much disclosed as possible. Then, it comes down to what anonymization technique are you going to use to do that? Are you going to generalize, are you going to pseudonymize, are you going to transform, are you going to use randomization, et cetera?
“And there are all these different techniques. Some of it is trial-specific, too. What kind of trial is it? If it’s pediatric, does age matter more than something else? If it’s weight loss, does weight matter more than something else? That’s where being able to do the ‘what if’ analysis or the scenarios around these trade-offs and still measuring to a maximum risk of re-identification comes into play and being able to do that quickly and effectively. Adverse events is another hot topic these days around making sure that as many of the AEs are disclosed as possible as well.”
6 Methods of Anonymization
Source: Wicks T (2023) Anonymizing Personal Data in Clinical Documents for CTIS Submission: Navigating the Privacy Tightrope
As the use of artificial intelligence (AI) continues to increase, one worry is its potential to compromise data privacy. “A systematic approach to evaluating risk of re-identification is even more important in this day where we have AI playing more of a role,” Zimmerman says.
“There is definite applicability of AI to help with identification of different attributes within a PDF document,” he adds. “So, take a CSR [clinical study report] or an IB [investigator’s brochure] to help identify different variables that need to be anonymized. But the anonymization techniques themselves, you need to have a tried-and-true approach for doing that, which means statistical analysis and understanding the trade-offs between anonymizing this attribute in this manner versus this other attribute a different way. What are the overall implications and how does that sum up to a total risk of re-identification overall for my subject population?”
3 Key Challenges in Anonymizing Clinical Submissions
Source: Kesari G (2022) How AI Is Helping Anonymize Clinical Trial Submissions. Forbes [Accessed Nov. 1, 2023].
Both Canada and the EMA strongly encourage quantitative anonymization of patient data, though they may accept qualitative anonymization. Zimmerman says with qualitative anonymization, organizations tend to have a static set of rules they apply across all their trials. Other personal data, for example, the names of study staff in CTIS submissions, other than the principal investigator or the person issuing the site suitability, may be redacted.
“The biggest takeaway with qualitative is it’s typically not measurable,” he says. “You don’t really know what your risk of re-identification is, and you don’t know what your data utility measures are. You need to be thinking ahead around, for example, the new anonymization report template from EMA with the relaunch of Policy 0070. They ask a lot of very specific questions, one of which is what is your residual risk and what was your risk of reidentification before and what’s the residual risk after your anonymization? And you’re going to struggle with a qualitative method to be able to answer some of those questions.
“Quantitative, on the other hand, is measurable, and … it’s a statistical analysis of the particular trial datasets to understand based on that patient population and all the identifiers and quasi-identifiers, what are the different rules that can be applied dynamically. So maybe for this trial tenure, age bands don’t make sense, and maybe 20-year age bands or five-year age bands make sense in order to maintain a particular risk threshold …. and optimize the data utility on the other end. … And what you get out of it is, it’s very measurable before what your risk of re-identification is. You’re able to explain to the regulator exactly what your data utility measures are as well.”
Greg Shear, disclosure services lead at ClaritiDox, says quantitative anonymization is something organizations will have to know about when submitting a marketing authorization through Policy 0070.
Figure 1: Methods of Data Anonymization Employed by Organizations
Source: Citeline survey data, July-October 2023
In terms of effective data anonymization strategies, Zimmerman notes not all trials are the same.
“You get into … maybe a rare disease trial that needs to be disclosed,” he says. “There are special considerations around rare diseases. There’s a very small patient population in your trial itself and across the globe. So, you need to give special consideration around how to anonymize, how to maximize data utility, realizing that you don’t have a big patient population to work with. Contrast that with a vaccines trial, [in] which you’re going to have tens of thousands of subjects and it’s a different kind of anonymization problem.
“In a rare disease situation and many other types of trials, when you’re working on a disclosure project, you need to be thinking about, for example, reference populations, what similar trials were run around the same time or overlapping with your trial that you’re working to anonymize, and how you can leverage the populations of those trials overlaid within your own to help increase some of the anonymization opportunities that you have, all while managing the risk of re-identification.”
Zimmerman says with the right tools, these techniques can be used and applied in statistical analyses. “Without tools, this stuff becomes very complex and very manual and error prone, and you certainly would need a statistician’s mindset to be able to do that,” he says. “But it’s going to take you an enormous amount of time and effort as opposed to having purpose-built tools that can do this systematically for you.”
“Definitely meeting that regulation and responsibly anonymizing that data, putting the documents out to the Health Canada and the EMA databases, is a great success in terms of trial transparency,” Shear says. “But to go beyond that, you’re already putting in this massive effort to anonymize these datasets. There’s plenty of initiatives out there that encourage data sharing like Vivli, Project Datasphere, and ClinicalStudyDataRequest.com [CSDR]. I think there are some big pharma companies with the YODA [Yale Open Data Access] Project and Duke Clinical Research Institute. [You’re] really taking this data that you’ve already put a lot of effort into collecting. And participants have participated in these trials to give you this data, and then really trying to increase the value of that by sharing it with other researchers. They can run further analysis. There’s a more public trust in terms of sharing full datasets.”
Shear says Policy 0070 has a second phase to be implemented at a later date that will include individual patient data. “So, [you’re] really looking at preparing for, first off, being able to maximize the use of this clinical data because it is valuable and sharing it with the scientific community, but then also preparing for the future, which is looking more like you’re going to have to share anonymized datasets either with health authorities or with other outside entities,” he says.
Figure 2: Organization’s Top Concerns in Data Anonymization
Source: Citeline survey data, July-October 2023
Vine says the consistency of anonymizing personal data within documentation is key. “From a data protection perspective, you are really looking at compliance with laws and regulations that have a significant amount of interplay with standards as set out in, for example, the EU’s GDPR [General Data Protection Regulation] with regards to the anonymization of personal data. Noncompliance could lead to action from regulators such as the EMA and data protection authorities worldwide as well as in respective EU member states. There’s a lot of publicity about actual and potential regulatory action under the GDPR and emerging privacy laws globally, so in addition to regulatory risk there is wider industry reputational risk coming from noncompliance to consider as well.
“There is always a re-identification risk and how that is managed by organizations is key, especially when considering acceptable re-identification probability thresholds under applicable regulations. It is important for organizations to regularly reassess the residual risk(s) of re-identification, re-evaluate these risks at a regular cadence, and regularly test the effectiveness of controls preventing re-identification and implement further controls if these do not suffice. A further consideration is the “over-anonymization” of submitted data. The reflection here is whether the level of anonymization affects the utility of the data being submitted, so not a re-identification risk, but rather one concerning the viability and usefulness of the data as a result of an anonymization. Organizations taking into consideration the impact of data transformations and redactions on the scientific and practical usefulness of the data is explicitly addressed in the relaunch of Policy 0070 as part of the anonymization report submission.”
Shear says these noncompliance issues could go beyond clinical trial regulation and into patient privacy regulations, “which have a lot more stringent penalties and a lot more capabilities for liability if you’re not anonymizing well.”
”When involving extraterritorial transfers of personal data in multinational trials, Vine says it’s best for the sponsor in question running the trial to “map and document where personal data is going to be transferred, to which geographic jurisdictions, which third-parties and/or vendors are involved in the transfer and the applicable legal and contractual safeguards and controls applied to the transfer.
“The GDPR Chapter V provisions for example, contain mechanisms by which organizations can transfer personal data relating to individuals in the Union to third countries” he says. “Adequacy is one possibility; however, there are others such as binding corporate rules or standard contractual clauses, which organizations can use as well.
“Cross-border data transfer restrictions can also be prevalent across other jurisdictions. If we take China and its recently enacted Personal Information Protection Law (PIPL), for example, the cross-border data transfer requirements can be more stringent than those of the EU, including requirements for the explicit consent of an individual for the transfer as well as a security assessment submitted to a relevant provincial authority for approval if the information is of a sensitive nature or of a high enough volume.
“The best course of action for organizations is to clearly map the personal data processing activity, the individuals to which the data relates and the destinations of the data pursuant to the transfer. Transfers on the basis of an intra-company arrangement as well as transfers to and involving third-party organizations and vendors need to be covered by the organization’s third-party risk assessment, due diligence and transfer impact assessment processes as well as the transfer (and wider vendor and third-party engagement) meeting all necessary contractual stipulations.”
5 Ways to Drive Continuous Improvement in Anonymization
Source: Wicks T (2023) Anonymizing Personal Data in Clinical Documents for CTIS Submission: Navigating the Privacy Tightrope